Team found AI-generated handoff notes to be accurate and thorough.
In recent research highlighted by JAMA Network Open, a team of scientists examined the potential of large language models (LLMs) to generate emergency medicine (EM) handoff notes. These notes, essential for patient care transitions, were evaluated for accuracy, safety, and practical use. The study explored whether integrating artificial intelligence could reduce the documentation load on physicians without jeopardizing patient outcomes. Conducted at a major New York hospital, the investigation analyzed over 1,600 EM patient encounters leading to hospital admissions.
While handoff notes play an important role in communication, they can also be prone to errors that can have serious consequences. Efforts to standardize these processes have gained traction, yet emergency medicine remains particularly challenging. Limited time, complex medical conditions, and diagnostic uncertainties often lead to inconsistencies. The introduction of electronic health record (EHR) tools has provided some relief, but emergency settings remain an area ripe for innovation.
LLMs, with their ability to process and summarize large amounts of data, present a promising avenue. However, concerns around their reliability and potential inaccuracies exist in all areas of healthcare and medicine. The study addressed these concerns by using a dual approach: fine-tuned LLMs complemented by rule-based heuristics. This method ensured that the notes followed established reporting standards while integrating essential clinical details.
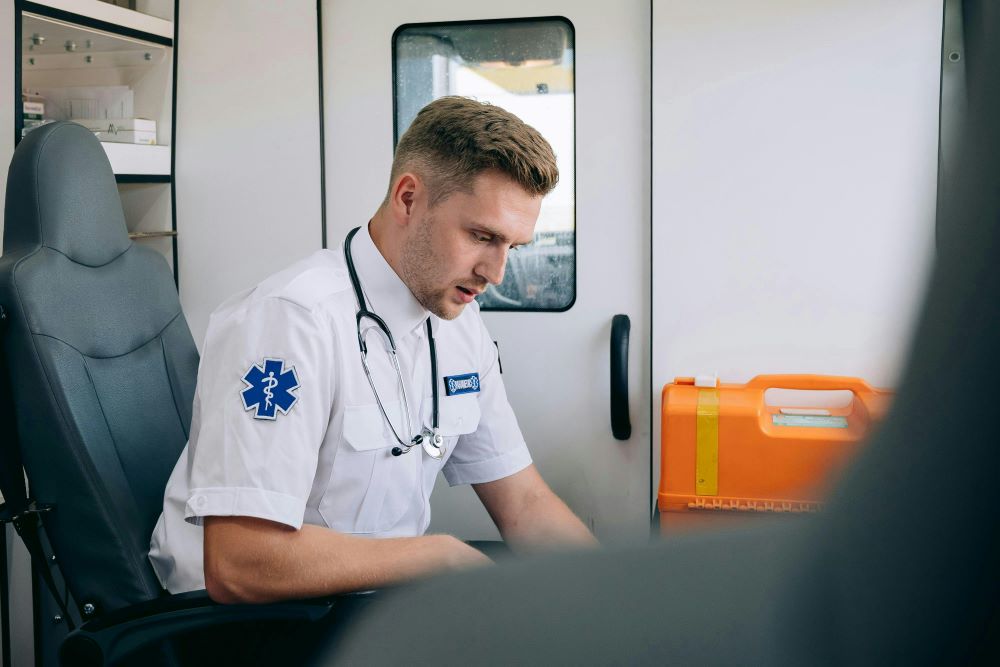
The template used for generating handoff notes mirrored traditional notes. Key patient data, such as laboratory results and vital signs, were included alongside narrative summaries of the patient’s history and potential diagnoses. Two LLMs, RoBERTa and Llama-2, were used for different aspects of the task, including identifying relevant content and crafting summaries. By excluding race-based information during model fine-tuning, the researchers hoped to minimize biases in the generated outputs.
Automated metrics and manual clinical evaluations formed the basis of the study’s analysis. Tools like ROUGE and BERTScore assessed lexical and semantic accuracy, while clinicians reviewed a sample of generated notes for completeness, clarity, and safety. Across 1,600 patient cases, the average patient age was 59.8 years, and women comprised 52% of the sample. Automated evaluations revealed that LLM-generated summaries often surpassed those written by physicians in terms of detail and alignment with source notes.
For example, ROUGE-2 scores for LLM-generated notes were significantly higher than those of physician-authored notes, reflecting superior text coverage and precision. Similarly, the SCALE approach, used to identify inconsistencies, highlighted fewer issues in AI-generated notes. Yet, the clinical reviews painted a more nuanced picture. While LLM-generated summaries were generally acceptable, they fell short of physician-written notes in areas like readability and contextual relevance. Potential safety risks, including incomplete information and flawed logic, were identified in 8-9% of cases, though none were deemed life-threatening.
The study also examined cases of hallucinated content, a well-documented limitation of AI systems. Interrater reliability assessments among clinicians indicated an agreement on criteria such as note correctness and usefulness, though readability ratings were less consistent.
Despite these limitations, the findings reveal the potential of LLMs to assist with medical documentation. By automating routine aspects of notetaking, these systems could allow physicians to focus more on direct patient care. The team highlighted the need for ongoing refinement to maintain these criteria.
Sources:
AI-generated handoff notes: Study assesses safety and accuracy in emergency medicine
Developing and Evaluating Large Language Model–Generated Emergency Medicine Handoff Notes
Join the conversation!