AI advancements are enhancing cancer imaging, automating tumor detection, and improving diagnostic accuracy.
Recent advancements in artificial intelligence are transforming the field of medical imaging, particularly in detecting and analyzing tumors through PET and CT scans, which have long been used for diagnosing cancer by identifying the size, location, and characteristics of tumors. PET scans reveal how tissues use energy, often with a radioactive form of glucose to highlight areas of abnormal activity indicating the presence of malignant tumors. CT scans, on the other hand, use X-rays to create detailed cross-sectional images of the body, helping pinpoint the exact location of these issues.
For cancer patients, imaging is often necessary to track multiple lesions caused by tumor growth. This manual process requires physicians to go through hundreds of images to identify and measure abnormalities, which is not only labor-intensive but also time-consuming. The introduction of AI-based algorithms is changing this landscape, offering ways to automate the evaluation process while maintaining or even enhancing accuracy.
In 2022, researchers from the Karlsruhe Institute of Technology (KIT) participated in an international competition aimed at improving tumor detection through artificial intelligence (AI). The event, known as autoPET, challenged teams to create algorithms capable of automatically identifying metabolically active tumor lesions in PET/CT images. The Karlsruhe team ranked among the top, showcasing a robust algorithm developed in collaboration with researchers from Essen’s Institute for Artificial Intelligence in Medicine.
The teams relied on advancements in deep learning techniques to analyze medical imaging data. Deep learning involves training artificial neural networks to recognize patterns in large datasets, enabling these systems to detect and classify complex features in imaging. For the autoPET challenge, researchers had access to a comprehensive dataset of annotated PET/CT scans, which they used to train their algorithms. The best-performing models employed ensemble methods—a combination of several algorithms—to improve detection accuracy and reliability.
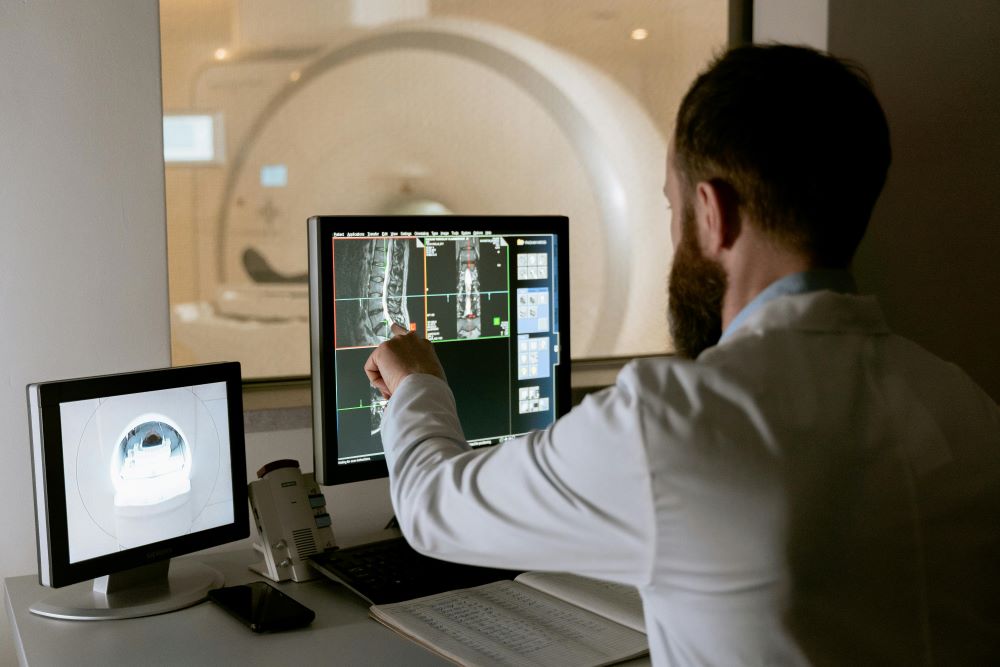
These systems have demonstrated an ability to identify tumor lesions quickly and with high precision. However, their performance still depends on the quality of training data and how the algorithms are designed. Researchers have highlighted the need for further development to ensure these tools perform consistently under varying conditions and can integrate seamlessly into clinical workflows. The ultimate goal is to fully automate the analysis of medical imaging data, freeing up time for medical professionals to focus on patient care.
AI advancements in imaging have the potential to significantly improve cancer diagnosis. By reducing the workload placed on physicians, who are already chronically overloaded, and increasing the speed and accuracy of imaging evaluations, these tools could lead to earlier interventions and better outcomes for patients. Still, much work remains to be done to refine these technologies, address potential biases in training data, and ensure the algorithms are robust enough for everyday clinical use.
The findings were recently published in the journal Nature Machine Intelligence, where researchers shared insights into the successes and challenges of developing these AI tools in general and their ability to revolutionize tumor detection, allowing medical teams to not only identify cancerous cells sooner but gain more precise insight into whether the cancer has spread. Computer-aided detection would also save time and allow for tailored treatments that can be established more quickly, which could lead to improvements in overall outcomes.
Sources:
New AI techniques improve tumor detection in PET and CT scans
Join the conversation!