University of Michigan researchers use machine learning to predict postoperative opioid dependency risks.
Researchers at the University of Michigan have made significant strides in predicting which patients are at risk of developing persistent opioid use after surgery. By leveraging machine learning algorithms, they hoped to discover a more tailored approach to postoperative care while addressing the ongoing public health crisis. Their findings, published in Plastic and Reconstructive Surgery, focus on using advanced data models to identify patients most vulnerable to long-term dependency.
The study involved training two distinct machine learning algorithms on different datasets. The first model, developed through the Michigan Genomics Initiative, incorporated both patient-reported data and clinical information, including individuals with or without prior opioid use. The second model relied on insurance claims data and was specifically designed for opioid-naive individuals—those with no prior history of opioid use before surgery. Both models were then tested to assess their ability to predict long-term opioid use among patients undergoing hand surgery.
Data from 889 patients who had hand surgery were analyzed for the study. Of these, 49 percent were opioid-naive, and 21 percent eventually developed persistent opioid use. Most of the surgeries fell into two categories: soft tissue procedures (55 percent) and fracture repair (20 percent). These insights were used to train and test the algorithms, revealing interesting contrasts between the two models.
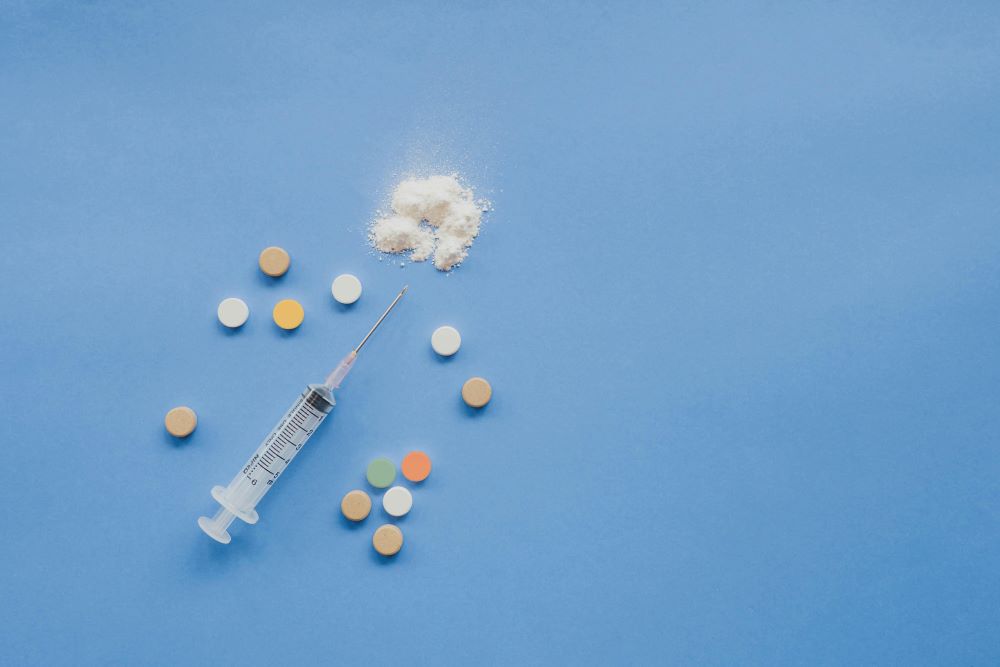
The Michigan Genomics Initiative model demonstrated strong predictive capabilities, achieving areas under the receiver operating curve (AUCs) of 0.84 and 0.85 when trained on hand surgery-specific data and a broader cohort of surgery patients, respectively. This indicates a high degree of accuracy in identifying those at risk. By contrast, the claims-based model performed less impressively, with AUCs of 0.69 for hand surgery patients and 0.52 for the broader group of opioid-naive surgery patients. These discrepancies highlight the importance of comprehensive, patient-centered data in developing effective predictive tools.
Persistent opioid use remains a significant concern in postoperative care. While opioids are critical for managing acute pain, extended use can lead to dependency, addiction, and other long-term health issues. Identifying patients most at risk before they leave the hospital is an important step in addressing and minimizing this challenge. Machine learning offers a promising solution, allowing clinicians to create personalized pain management strategies that reduce the likelihood of prolonged opioid use while maintaining sufficient pain control.
By integrating machine learning into clinical workflows, physicians could potentially reduce the burden of making complex, individualized pain management decisions. These tools could streamline the process of identifying at-risk patients, enabling healthcare teams to intervene earlier with alternative therapies or closer monitoring.
However, the study also highlights challenges that remain in adopting machine learning broadly in healthcare. For example, disparities in data availability, differences in surgical practices, and varying patient populations can all influence the generalizability of predictive models. Addressing these challenges will require ongoing collaboration between researchers, clinicians, and policymakers.
Despite these obstacles, the potential for machine learning to revolutionize postoperative care is clear. As healthcare systems continue to evolve, integrating advanced technologies like these could pave the way for more personalized, efficient, and effective treatment plans that limit long-term pain management side effects and addiction.
Sources:
Machine Learning Can ID Risk for Persistent Opioid Use After Surgery
Predicting Persistent Opioid Use after Hand Surgery: A Machine Learning Approach
Join the conversation!