The use of Generative AI for even straightforward tasks like the eDiscovery example cited above is still very modest.
Compiling relevant documentation has long been a rite of passage for young attorneys. Days and often nights are spent trawling through hundreds or even thousands of documents to help narrow down evidence for more senior colleagues to build a company’s defense in a litigation case. But things are changing, and young attorneys are increasingly being spared from this monotonous work.
Generative AI has advanced to the stage where it can undertake this very specific work to a similar or higher standard than humans. Let me be clear: I do not believe technology will replace highly trained attorneys in the foreseeable future, but for specific tasks like deciding if a document is relevant to a case, Generative AI is already proving its worth.
Imagine a well-known company receiving a harassment filing from several former employees relating to the firm’s CEO and a general culture of sexual harassment. The first task for the in-house legal team is to amass any data or documentation the company has relating to those plaintiffs and the CEO, as well as anything that may uncover harassment. Legal counsel must advise the company if it should defend or settle the case.
This material may include any messages exchanged between relevant individuals across company platforms like email, Slack, or Microsoft Teams. It may also include HR documents or messages exchanged between the plaintiffs and the HR department. Using traditional document discovery methods, it’s not unusual for a company to identify millions of individual messages that must be checked for relevance. Enter the young attorneys with a large supply of coffee and no weekend plans.
Here, Generative AI can digest the initial filing and then read each of the potentially relevant documents and messages contained in the company’s systems to form a judgment on whether they’re relevant. This is where previous generations of technology have failed.
While we’ve been able to search for keywords, like email exchanges between the plaintiffs and the CEO or Slack messages exchanged at the company containing sexually explicit language, it’s only now that AI can determine if those exchanges are relevant or irrelevant to the specific harassment case.
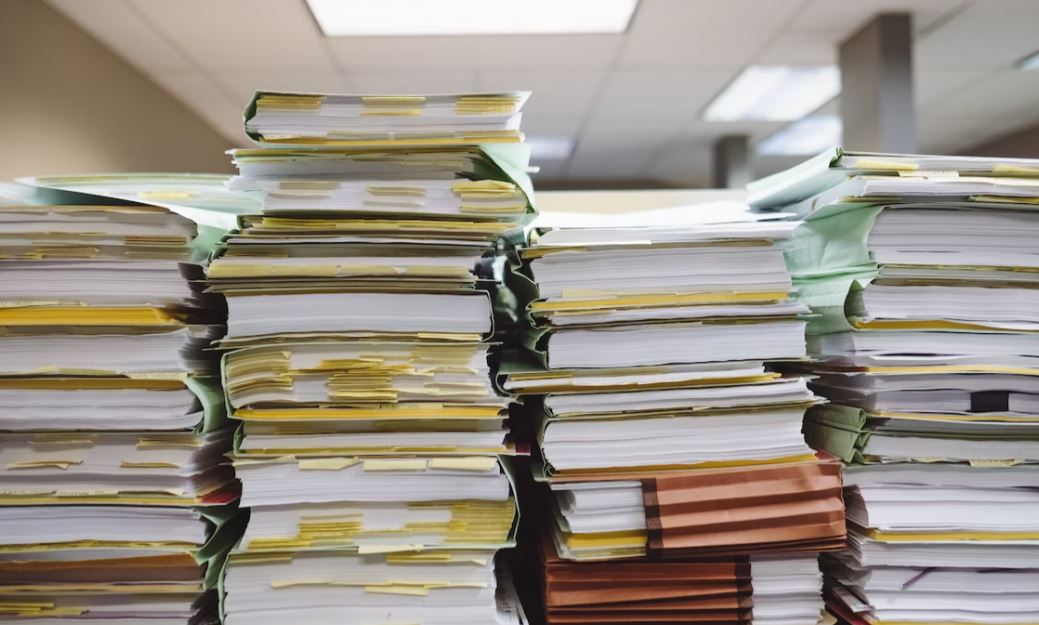
Harassment cases can be subtle. The language contained within messages may not be overt, but it may still be relevant. For example, “I wonder if plaintiff X can make my number go up.” Previous generations of technology would have missed this type of exchange, but large language models (LLMs) can appreciate subtext just like humans, and this message would be flagged for review.
What does all this mean for the future of litigation? Aside from freeing young attorneys for more meaningful work, the introduction of AI means we’re likely to see significantly faster responses to litigation. It means in-house legal teams and their supporting legal firms will be able to do more with less and focus on advising the company rather than reviewing documents. It doesn’t mean we will have chatbots that can offer legal advice any time soon.
The use of Generative AI for even straightforward tasks like the eDiscovery example cited above is still very modest. To date, the cost has been prohibitive for all but the largest firms responding to the most significant litigation. However, this is beginning to change as legal tech firms realize Chat GPT is too expensive, and smaller language models can get the job done much more cost-effectively. So, while there’s no need for attorneys to fear job displacement, it’s important for firms and in-house litigation teams to begin experimenting with this new capability.
Join the conversation!